The standalone DAVIS initiative is in maintenance mode: we won't be hosting any more DAVIS challenges and we will no longer update the benchmark leaderboards on this page. We have integrated the existing results into paperswithcode and you can enter the results for you latest paper in their corresponding task web pages:
Also, we will continue running the evaluation servers in Codalab. We would like to thank the community for taking part in the challenges and we encourage everyone to keep using the datasets for video object segmentation or any other task!Datasets
- DAVIS 2016: In each video sequence a single instance is annotated.
- DAVIS 2017 Semi-supervised: In each video sequence multiple instances are annotated.
- DAVIS 2017 Unsupervised: In each video sequence multiple instances are annotated.
Semi-supervised and Unsupervised refer to the level of human interaction at test time, not during the training phase. In Semi-supervised, better called human guided, the segmentation mask for the objects of interest is provided in the first frame. In Unsupervised, better called human non-guided, no human input is provided.
Publications
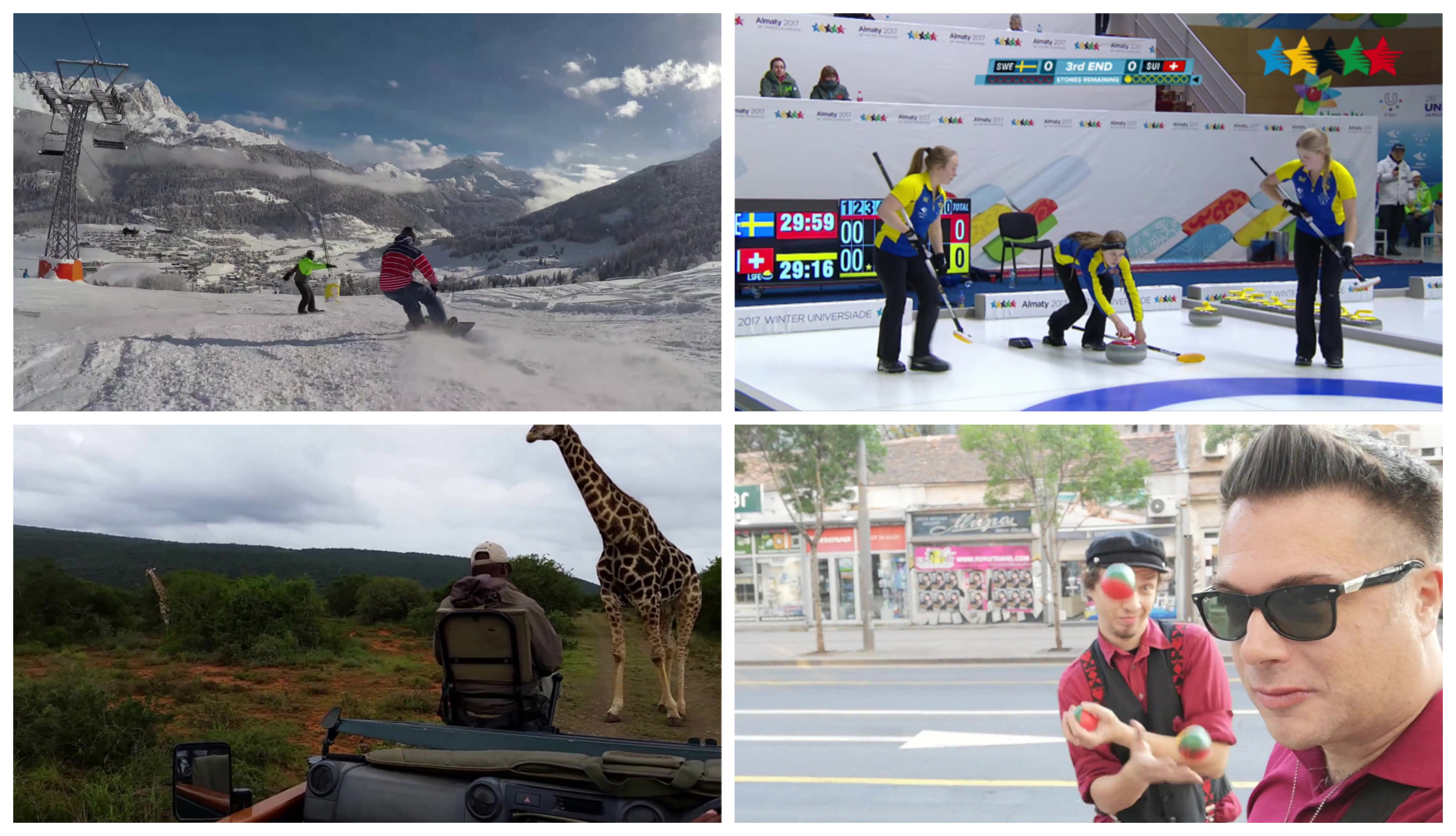
The 2019 DAVIS Challenge on VOS: Unsupervised Multi-Object Segmentation
S. Caelles,
J. Pont-Tuset,
F. Perazzi,
A. Montes,
K.-K. Maninis, and
L. Van Gool
arXiv:1905.00737, 2019
[PDF] [BibTex]
@article{Caelles_arXiv_2019, author = {Sergi Caelles and Jordi Pont-Tuset and Federico Perazzi and Alberto Montes and Kevis-Kokitsi Maninis and Luc {Van Gool}}, title = {The 2019 DAVIS Challenge on VOS: Unsupervised Multi-Object Segmentation}, journal = {arXiv:1905.00737}, year = {2019} }
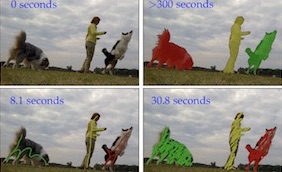
The 2018 DAVIS Challenge on Video Object Segmentation
S. Caelles,
A. Montes,
K.-K. Maninis,
Y. Chen,
L. Van Gool,
F. Perazzi, and
J. Pont-Tuset
arXiv:1803.00557, 2018
[PDF] [BibTex]
@article{Caelles_arXiv_2018, author = {Sergi Caelles and Alberto Montes and Kevis-Kokitsi Maninis and Yuhua Chen and Luc {Van Gool} and Federico Perazzi and Jordi Pont-Tuset}, title = {The 2018 DAVIS Challenge on Video Object Segmentation}, journal = {arXiv:1803.00557}, year = {2018} }
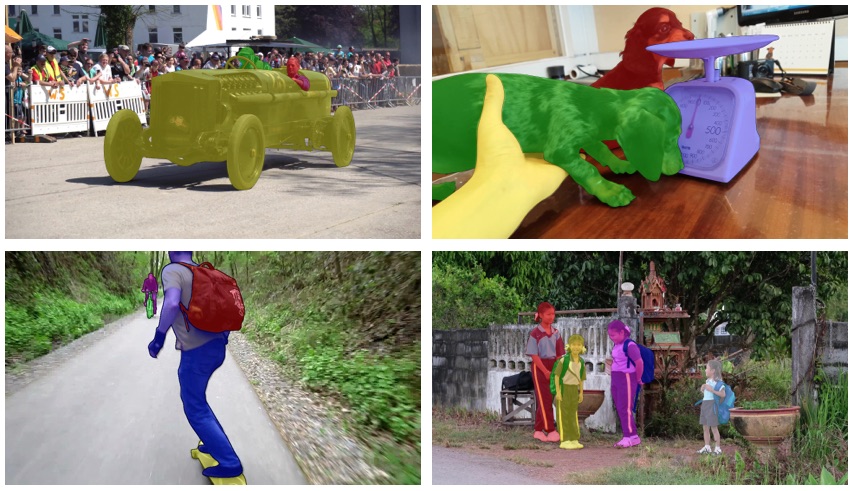
The 2017 DAVIS Challenge on Video Object Segmentation
J. Pont-Tuset,
F. Perazzi,
S. Caelles,
P. Arbeláez,
A. Sorkine-Hornung, and
L. Van Gool
arXiv:1704.00675, 2017
[PDF] [BibTex]
@article{Pont-Tuset_arXiv_2017, author = {Jordi Pont-Tuset and Federico Perazzi and Sergi Caelles and Pablo Arbel\'aez and Alexander Sorkine-Hornung and Luc {Van Gool}}, title = {The 2017 DAVIS Challenge on Video Object Segmentation}, journal = {arXiv:1704.00675}, year = {2017} }
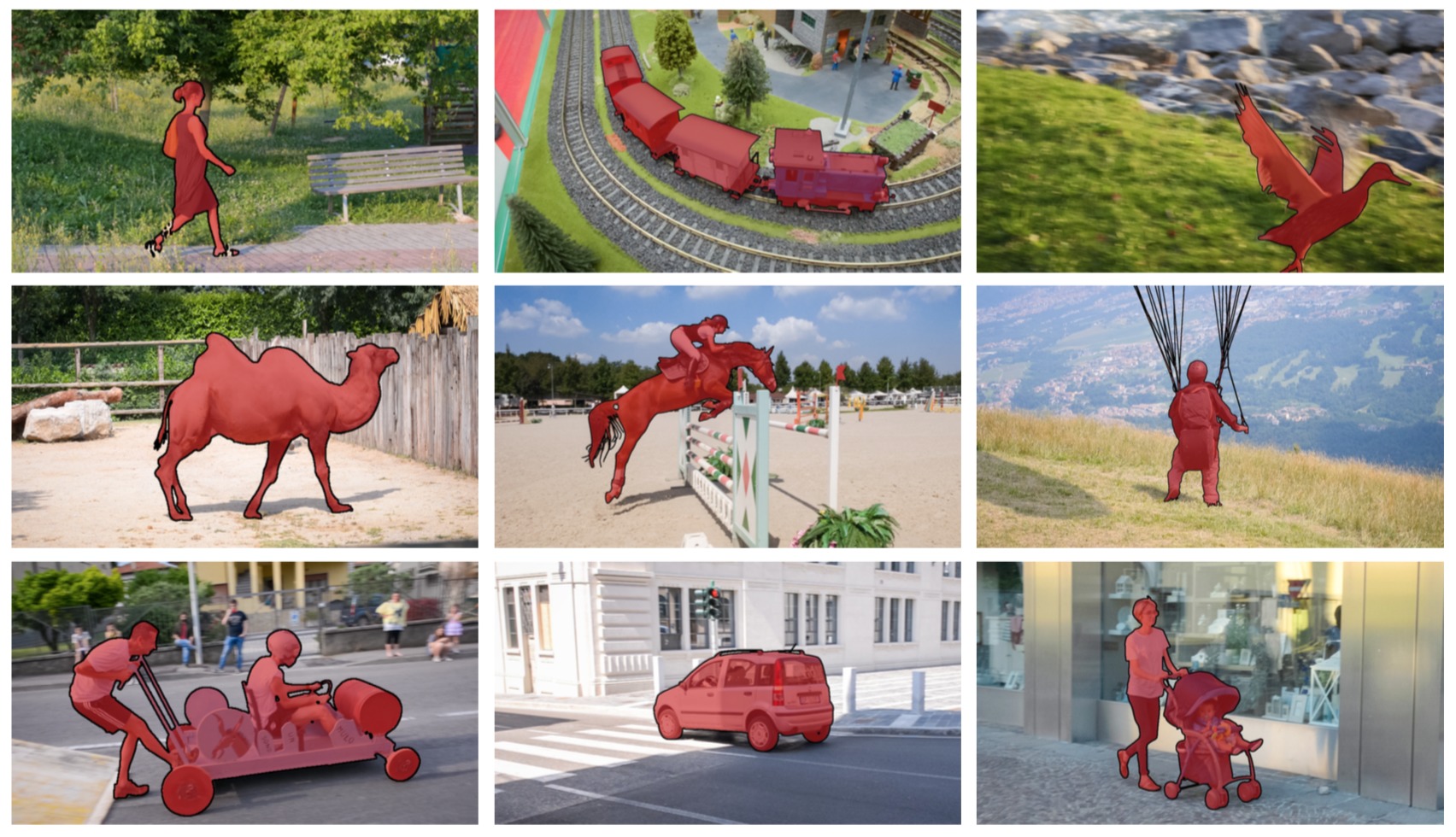
A Benchmark Dataset and Evaluation Methodology for Video Object Segmentation
F. Perazzi,
J. Pont-Tuset,
B. McWilliams,
L. Van Gool,
M. Gross, and
A. Sorkine-Hornung
Computer Vision and Pattern Recognition (CVPR) 2016
[PDF] [Supplemental] [BibTex]
@inproceedings{Perazzi2016,
author = {F. Perazzi and J. Pont-Tuset and B. McWilliams and L. {Van Gool} and M. Gross and A. Sorkine-Hornung},
title = {A Benchmark Dataset and Evaluation Methodology for Video Object Segmentation},
booktitle = {Computer Vision and Pattern Recognition},
year = {2016}
}
Preview of the Annotations (Train and Val DAVIS 2017 Semi-supervised)
Benchmark State-of-the-Art
Display the evaluation of the current State-of-the-Art segmentation tecniques in DAVIS; using the three presented measures in our work.
Explore State-of-the-Art Results
Visualize the segmentation results for all state-of-the-Art techniques on all DAVIS 2016 images, right from your browser.
Downloads
Download the DAVIS images and annotations, pre-computed results from all techniques, and the code to reproduce the evaluation.
Contributions from the community
These are the papers and projects in which the community has augmented our datasets:
-
Referring expression annotations for DAVIS 2016 and DAVIS 2017
It contains the referring expression given the first frame to two annotators in DAVIS 2016 and DAVIS 2017. Moreover, it contains the referring expression given the whole video to two annotators in DAVIS 2017.Video Object Segmentation with Language Referring Expressions
A. Khoreva, A. Rohrbach, and B. Schiele
ACCV 2018
[Website] [BibTex]@inproceedings{KhoRohrSch_ACCV2018, title = {Video Object Segmentation with Language Referring Expressions}, author = {Khoreva, Anna and Rohrbach, Anna and Schiele, Bernt}, year = {2018}, booktitle = {ACCV} }
-
Human eye fixation for DAVIS 2016.
It contains human eye fixation data captured with professional equipment by twenty participants for all the frames in DAVIS 2016 Train and Val.@inproceedings{Wang_2019_CVPR, title = {Learning Unsupervised Video Object Segmentation through Visual Attention}, author = {Wang, Wenguan and Song, Hongmei and Zhao, Shuyang and Shen, Jianbing and Zhao, Sanyuan and Hoi, Steven Chu Hong and Ling, Haibin}, year = {2019}, booktitle = {CVPR} }
-
Shadow annotations for DAVIS 2016.
It contains the annotation of object shadows in 29 videos of DAVIS 2016.@inproceedings{Huang_2016, title = {Temporally Coherent Completion of Dynamic Video}, author = {Huang, Jia-Bin and Kang, Sing Bing and Ahuja, Narendra and Kopf, Johannes}, year = {2016}, booktitle = {ACM} }