Definition
The semi-supervised scenario assumes the user inputs a full mask of the object of interest in the first frame of a video sequence. Methods have to produce the segmentation mask for that object in the subsequent frames.More information in the DAVIS 2017 publication and the Codalab submission site.
Dates and Phases
- Test-Dev: Unlimited number of submissions, open end.- Test-Challenge: Limited to 5 submissions in total from 12th May 2019 23:59 UTC to 24th May 2019 23:59 UTC.
Prizes
The winner of the competition will get an NVIDIA GEFORCE RTX 2080Ti.All the other participants invited to the workshop will get a subscription to Adobe CC for 1 year.
Submission
New submission site!Submissions to all phases are done through the new Codalab site of the challenge (different from previous editions). We have speeded up the evaluation time by a factor of 5!
Please register to the site and read carefully the instructions in the Learn the Details -> Overview and Evaluation sections.
Datasets (Download here, 480p resolution)
- Train + Val: 90 sequences from DAVIS 2017.- Test-Dev 2017: 30 sequences from DAVIS 2017 Semi-supervised. Ground truth not publicly available.
- Test-Challenge 2017: 30 sequences from DAVIS 2017 Semi-supervised. Ground truth not publicly available.
Feel free to train or pre-train your algorithms on any other dataset apart from DAVIS (Youtube-VOS, MS COCO, Pascal, etc.) or use the full resolution DAVIS annotations and images.
Evaluation
- The per-object measures are those described in the original DAVIS CVPR 2016 paper: Region Jaccard (J) and Boundary F measure (F).- The overall ranking measures are computed as the mean between J and F, both averaged over all objects. Precise definitions available in the papers DAVIS 2016 and DAVIS 2017 publications.
Papers
- Right after the Challenge closes (24th May) we will invite all participants to submit a short abstract (400 words maximum) of their method (Deadline 29th of May, 23:59 UTC).- Together with the results obtained, we will decide which teams are accepted at the workshop. Date of notification June 3rd.
- Accepted teams will be able to submit a paper describing their approach (Deadline 12th June, 23:59 UTC). The template of the paper is the same as CVPR, but length will be limited to 4 pages including references.
- Papers will also be invited to the workshop in form of oral presentation or poster.
- Accepted papers will be self-published in the web of the challenge (not in the official proceedings, although they have the same value).
Other considerations
- Each entry must be associated to a team and provide its affiliation.- The best entry of each team will be public in the leaderboard at all times.
- We will only consider the "semi-supervised" scenario: the mask of the first frame is given, no human interaction/refinement allowed. Even though the class of each object was recently released, it should not be used as additional information in the methods. Although we have no way to check the latter at the challenge stage, we will make our best to detect it a posteriori before the workshop.
- We reserve the right to remove one of the entry methods to the competition when there is a high technical similarity to methods published in previous conferences or workshops. We do so in order to keep the workshop interesting and to push state of the art to move forward.
- The new annotations in this dataset belong to the organizers of the challenge and are licensed under a Creative Commons Attribution 4.0 License.
Citation
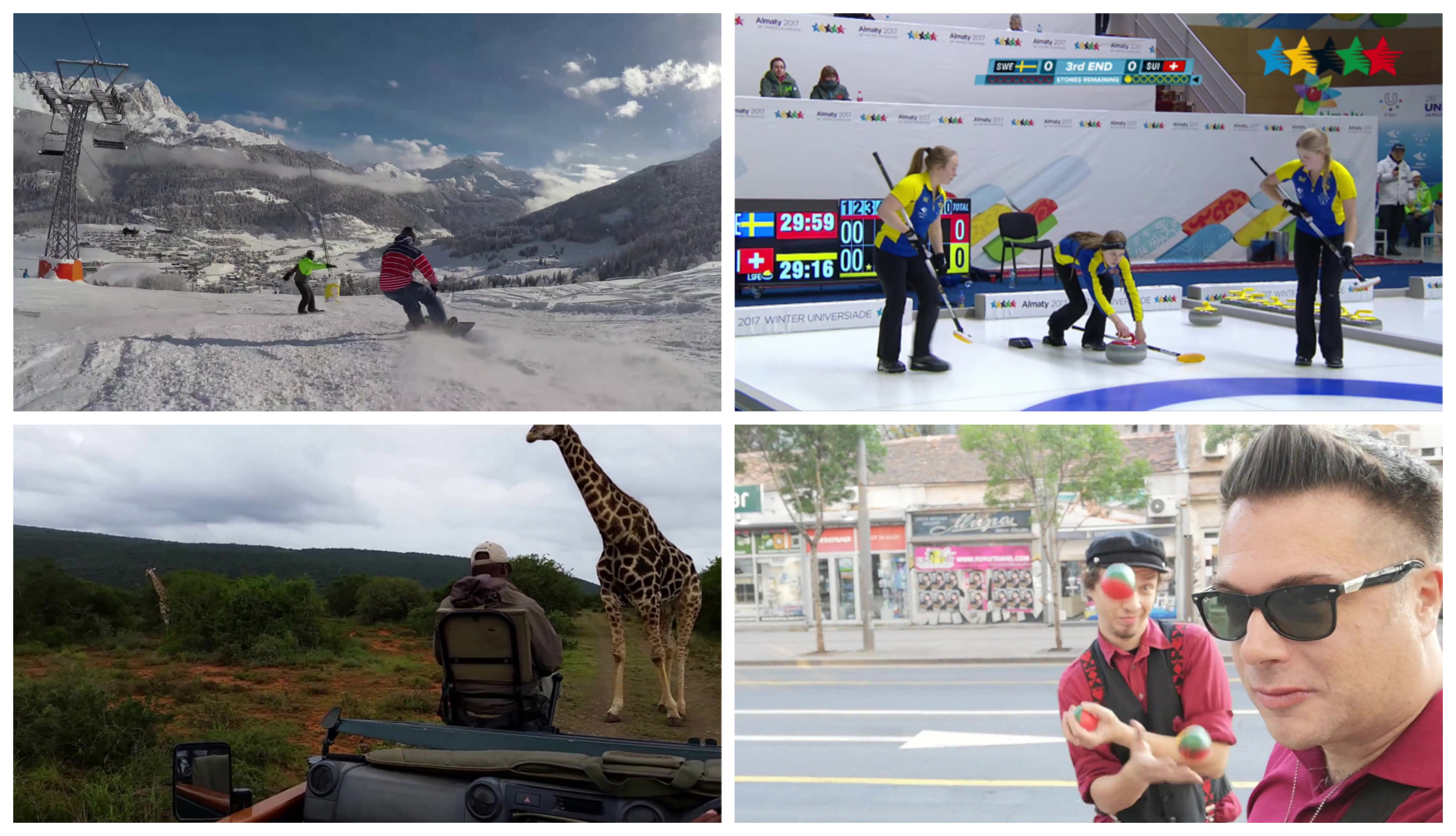
The 2019 DAVIS Challenge on VOS: Unsupervised Multi-Object Segmentation
S. Caelles,
J. Pont-Tuset,
F. Perazzi,
A. Montes,
K.-K. Maninis, and
L. Van Gool
arXiv:1905.00737, 2019
[PDF] [BibTex]
@article{Caelles_arXiv_2019, author = {Sergi Caelles and Jordi Pont-Tuset and Federico Perazzi and Alberto Montes and Kevis-Kokitsi Maninis and Luc {Van Gool}}, title = {The 2019 DAVIS Challenge on VOS: Unsupervised Multi-Object Segmentation}, journal = {arXiv:1905.00737}, year = {2019} }
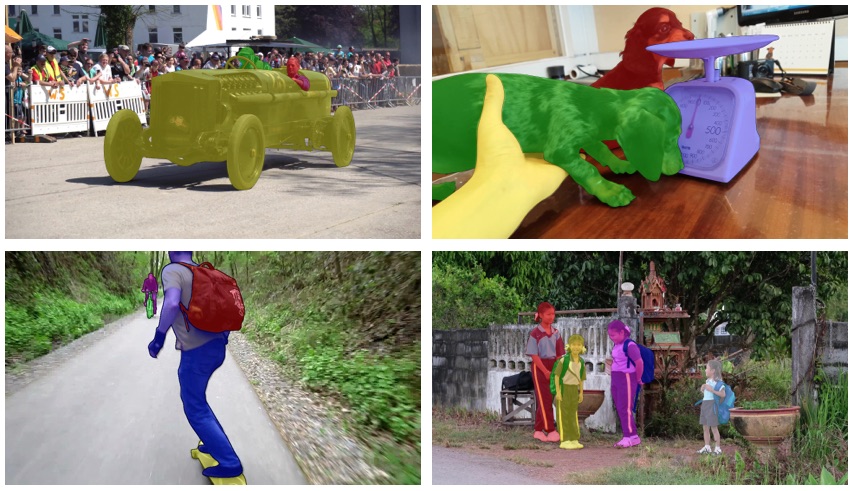
The 2017 DAVIS Challenge on Video Object Segmentation
J. Pont-Tuset,
F. Perazzi,
S. Caelles,
P. Arbeláez,
A. Sorkine-Hornung, and
L. Van Gool
arXiv:1704.00675, 2017
[PDF] [BibTex]
@article{Pont-Tuset_arXiv_2017, author = {Jordi Pont-Tuset and Federico Perazzi and Sergi Caelles and Pablo Arbel\'aez and Alexander Sorkine-Hornung and Luc {Van Gool}}, title = {The 2017 DAVIS Challenge on Video Object Segmentation}, journal = {arXiv:1704.00675}, year = {2017} }
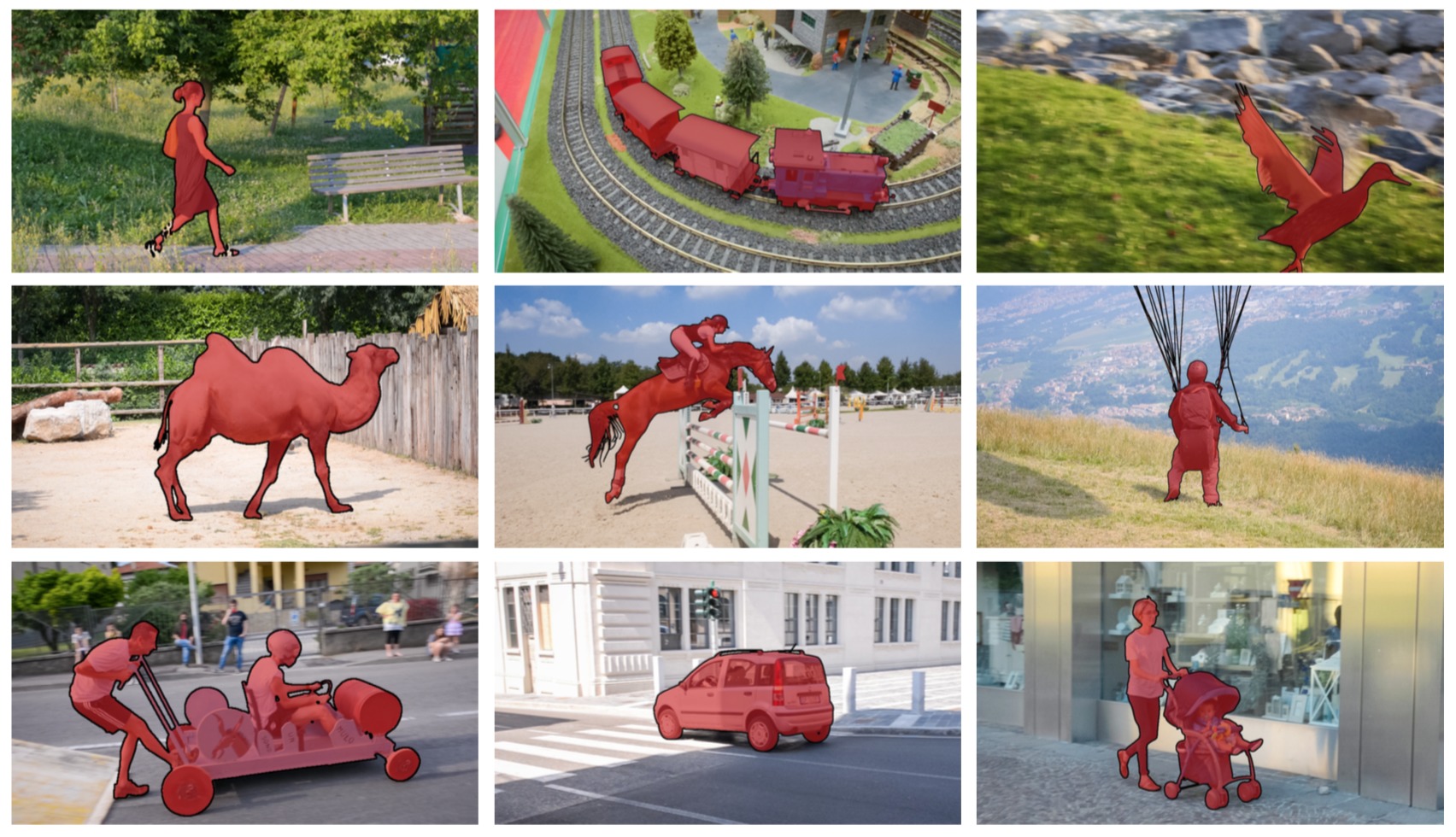
A Benchmark Dataset and Evaluation Methodology for Video Object Segmentation
F. Perazzi,
J. Pont-Tuset,
B. McWilliams,
L. Van Gool,
M. Gross, and
A. Sorkine-Hornung
Computer Vision and Pattern Recognition (CVPR) 2016
[PDF] [Supplemental] [BibTex]
@inproceedings{Perazzi2016,
author = {F. Perazzi and J. Pont-Tuset and B. McWilliams and L. {Van Gool} and M. Gross and A. Sorkine-Hornung},
title = {A Benchmark Dataset and Evaluation Methodology for Video Object Segmentation},
booktitle = {Computer Vision and Pattern Recognition},
year = {2016}
}